Explorative Gradient Method (EGM) --- a solution to high-dimensional parameter space
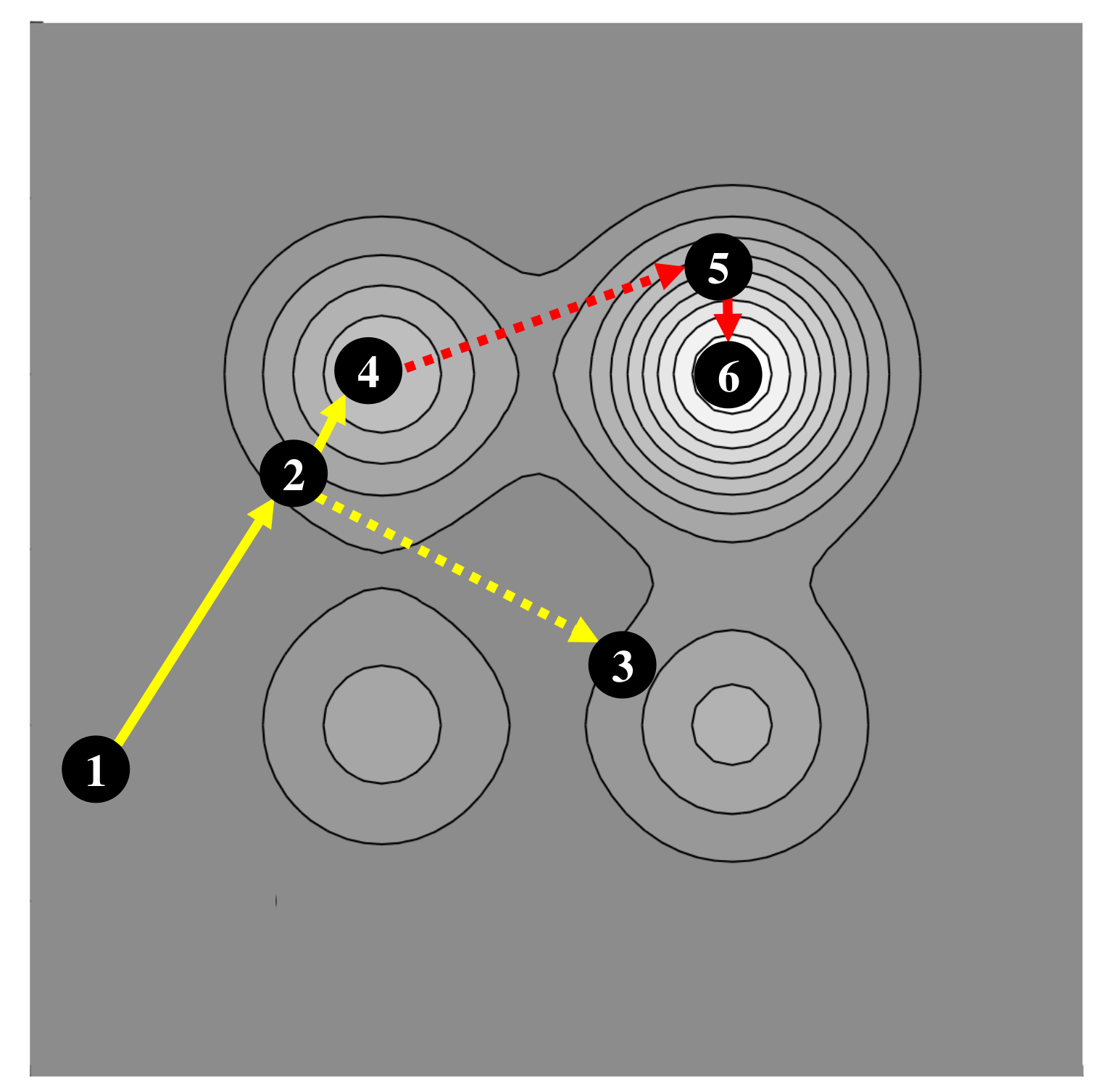
This method is developed by Yiqing Li and friends. A distinguishing feature of EGM is that it performs an ‘aggressive’ exploitation in one step (the solid lines) and the arguably most optimal exploration in another step (the dash lines). See Journal of Fluid Mechanics, 932, A7 for more details.
gradient-enriched Machine Learning Control (gMLC) --- a solution to MIMO control law space
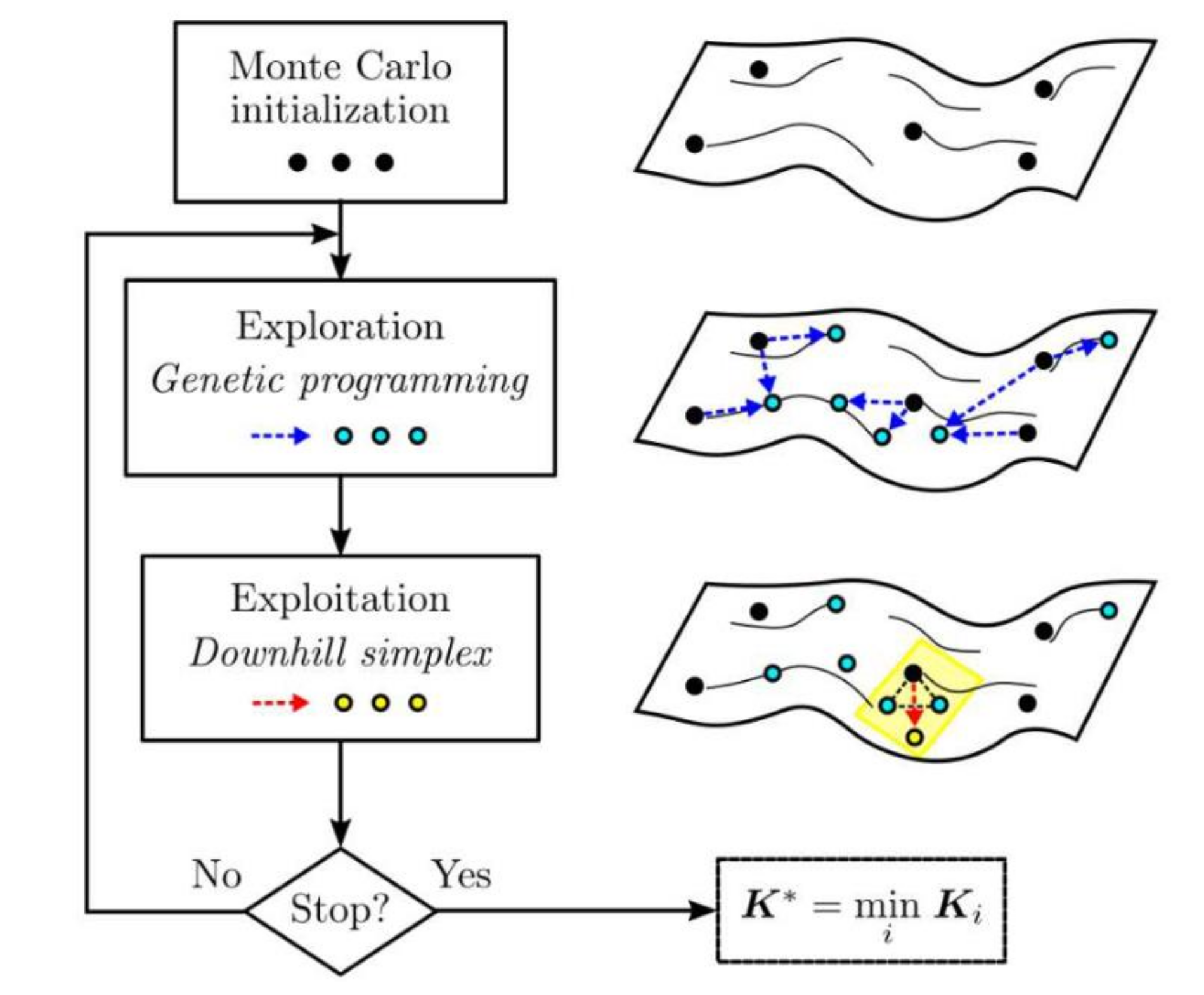
This method is developed by Guy Y. Cornejo Maceda, Yiqing Li and friends. The key idea of EGM is extended to the function space. In the proposed gradient-enriched MLC, the exploitation in MLC is accelerated by downhill subplex iteration. See Journal of Fluid Mechanics, 917, A42 for more details.
Bayesian optimization (BO) --- a solution to design and optimization of black-box functions
Antoine Blanchard and Themis Sapsis develop new criteria that guide the algorithm towards highly anomalous—and therefore highly relevant—regions of the search space. See Journal of Computational Physics 425, 109901 (2021) for more details.